AI in Medical Coding: Overcoming Billing Inefficiencies in Healthcare
Medical coding and billing inefficiencies cost healthcare providers time and revenue. Issues like missed charges, coding errors, and slow claim processing lead ...
January 07, 2025
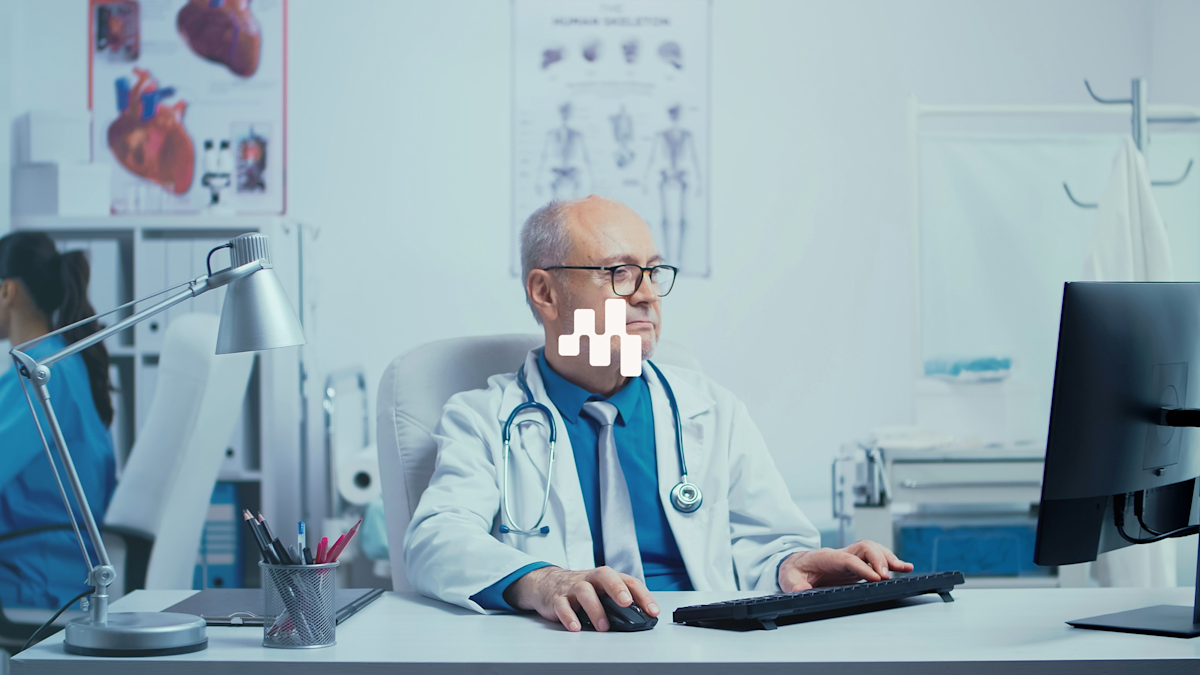
Medical coding and billing inefficiencies cost healthcare providers time and revenue. Issues like missed charges, coding errors, and slow claim processing lead to high denial rates and delayed reimbursements. This article examines the scope of these problems and how AI-driven solutions – including machine learning (ML) and natural language processing (NLP) – can improve billing efficiency and healthcare revenue cycle management. We’ll also explore real-world results from automated medical coding deployments, challenges to AI adoption (EHR integration, data privacy, and compliance), and market trends for AI in revenue cycle management (RCM).
Missed Billing Opportunities and Claim Denial Rates
Manual coding processes often result in missed billing (lost charges) and high rates of claim rejections/denials. Industry data shows that:
Nearly 20% of all medical claims are denied on first submission ( Claims Denials: A Step-by-Step Approach to Resolution ). In some insurer segments, denial rates average 10% or more and have been rising (over 20% increase in five years) ( Claims Denials: A Step-by-Step Approach to Resolution ).
Coding errors and omissions contribute significantly to denials. Research finds insurers typically reject 10–20% of claims they receive (Stop living in denials – The impact of inefficient billing processes), frequently due to issues like missing information or incorrect coding.
Missed charges are a hidden revenue leak. Up to 1% of a health system’s net charges can be lost due to poor charge capture processes (Charge Capture Optimization: Target Five Hotspots to Boost the Bottom Line). These are services provided but never billed, often because of documentation or coding gaps.
High denial and rejection rates not only mean potential revenue loss, but they also incur extra labor to appeal or correct claims. Unfortunately, as many as 60% of denied claims are never resubmitted by providers ( Claims Denials: A Step-by-Step Approach to Resolution ), meaning the revenue is written off. In other words, when billing processes fail, healthcare organizations leave money on the table.
Delays in Claim Processing and Reimbursement
Inefficient billing workflows lead to delays in claims processing and slower reimbursement cycles for clinics and hospitals. Key indicators of these delays include:
Long accounts receivable times: Experts recommend keeping average days in A/R under ~35 days and having <10% of claims over 90 days old (What Is Accounts Receivable in Healthcare | A/R Explained). In reality, many providers struggle with far longer timelines. A recent analysis showed 31% of inpatient claims to commercial payers remained unpaid after 90 days (vs. 12% for Medicare claims) (Commercial payers delay paying out claims to providers | Healthcare Finance News). Outpatient claims showed a similar gap, with about one-third taking over 3 months to pay (Commercial payers delay paying out claims to providers | Healthcare Finance News).
Extended payment cycles: Complex authorization and claim reviews can drag out payments. Certain denial types (e.g. requests for more information) automatically delay payments by 45+ days (Commercial payers delay paying out claims to providers | Healthcare Finance News). Each extra day in A/R negatively impacts cash flow and increases the risk of non-payment.
Administrative bottlenecks: Clinics often face backlogs in claim submission or error resolution, further stalling reimbursement. An inefficient billing process can mean frequent resubmissions and appeals, prolonging the revenue cycle. In one survey, 50% of patients in hospitals experienced delayed discharge due to slow insurance claim processing, underscoring how billing delays impact operations as well (Slow claim processing delays patient-discharge — Study).
Overall, delayed reimbursement strains healthcare finances. When payments take weeks or months, organizations must cover operational costs without incoming revenue, which can threaten financial stability – especially for smaller practices or those with tight margins.
Financial Impact of Manual Coding Inefficiencies
The financial toll of manual coding inefficiencies is significant, affecting both revenue and operational costs. Consider these findings on how coding and billing issues hit the bottom line:
Hospitals lose an estimated $5 million annually on average (around 5% of net patient revenue) due to unresolved claim denials ( Claims Denials: A Step-by-Step Approach to Resolution ). Each denied claim that isn’t recovered represents services provided with no payment.
The cost to rework denials is high. It costs about $25 for a physician practice to reprocess a denied claim, and $181 per claim for a hospital ( Claims Denials: A Step-by-Step Approach to Resolution ). This is pure waste spent on back-end fixes that efficient coding could avoid.
Providers collectively waste $10.6 billion per year fighting claims denials ( The True Costs of Coding Errors: How AI Turns Challenges Into Opportunities ) ( The True Costs of Coding Errors: How AI Turns Challenges Into Opportunities ). This figure (from a Premier health survey) includes labor for denial management and appeals. It’s part of the broader administrative overhead, with the U.S. healthcare system spending over $82.7 billion on administrative tasks in 2022 ( The True Costs of Coding Errors: How AI Turns Challenges Into Opportunities ).
Coding-related revenue loss is substantial. The AMA found 11% of all claims were denied in 2022, equating to roughly 110,000 unpaid claims for an average health system ( The True Costs of Coding Errors: How AI Turns Challenges Into Opportunities ). Many of these denials trace back to coding errors or insufficient documentation. Moreover, chronic under-coding (failing to bill for the full extent of care) means providers simply never bill for some services, directly missing out on earned revenue ( The True Costs of Coding Errors: How AI Turns Challenges Into Opportunities ).
Once claims are denied or delayed, reimbursement often drops. Industry research shows 65% of denied claims are never corrected and resubmitted by providers (Stop living in denials – The impact of inefficient billing processes), resulting in permanent revenue loss. Even when corrected, delayed payments may only be partially reimbursed, or counted as bad debt if too aged.
In summary, manual coding inefficiencies create a cascade of financial issues: lost charges, high denial write-offs, increased labor costs, and disrupted cash flow. This has driven healthcare organizations to seek solutions that can tighten the revenue cycle and capture revenue more effectively.
AI-Driven Solutions for Coding Accuracy and Billing Efficiency
Artificial intelligence is increasingly seen as a game-changer for medical coding and billing. AI-driven solutions – including machine learning coding systems and NLP in healthcare coding – can dramatically improve accuracy and efficiency in several ways:
Automated medical coding: AI algorithms (trained on millions of records) can scan clinical documentation and suggest or assign codes automatically. NLP technology interprets free-text doctors’ notes and identifies diagnoses, procedures, and relevant keywords to match them with the correct ICD-10 or CPT codes (Technological Advances in Medical Coding) (Technological Advances in Medical Coding). For example, if a note says "acute bronchitis," an AI coder can recognize that phrase and assign the corresponding code without manual lookup (Technological Advances in Medical Coding). Routine cases can be coded end-to-end by AI, freeing human coders to focus on complex cases.
Improved coding accuracy: Machine learning models learn from historical coding corrections to reduce errors. AI systems apply coding rules consistently across all records, eliminating the variability of human coders. They also stay up-to-date with code updates. This leads to fewer mistakes like up-coding or under-coding. In practice, hospitals using AI-assisted coding have seen accuracy improvements of over 20% (How AI is Improving Medical Coding Accuracy and Efficiency | Medwave), directly reducing claim rejections. Consistent, thorough coding also means more complete billing (capturing all billable services), which increases appropriate reimbursement (How AI is Improving Medical Coding Accuracy and Efficiency | Medwave).
Real-time error checking: AI acts as a diligent second pair of eyes, performing real-time auditing of codes and claims. It can instantly flag missing information or likely coding errors before a claim is submitted (How AI is Improving Medical Coding Accuracy and Efficiency | Medwave). For instance, if a procedure code is entered without a required modifier or if documentation doesn’t support a billed code, the AI system can warn staff immediately. Such AI-driven claim scrubbing prevents common mistakes that lead to denials (e.g. incompatible codes, lacking authorization, or medical necessity issues), thereby increasing first-pass claim acceptance.
Faster billing workflows: Automating labor-intensive steps speeds up the revenue cycle. AI coding tools can process charts much faster than humans – some hospitals report a 30% reduction in coding time after AI implementation (How AI is Improving Medical Coding Accuracy and Efficiency | Medwave). This means claims get out the door faster. Additionally, AI-driven claim routing can automatically submit clean claims or route problematic ones for human review. Overall, practices have achieved a 25% faster turnaround in billing using AI coding assistance (How AI is Improving Medical Coding Accuracy and Efficiency | Medwave). Shorter coding and billing cycles translate to quicker reimbursements and improved cash flow.
Adaptive learning and insights: Beyond automation, AI provides analytics to continuously improve RCM. Machine learning models can analyze denial patterns to predict and prevent future denials. For example, if certain procedure codes are frequently denied by a payer, the AI can alert coders or even preemptively adjust coding (within compliant bounds) to meet payer requirements (Stop living in denials – The impact of inefficient billing processes) (Stop living in denials – The impact of inefficient billing processes). Some AI platforms also offer financial forecasting, using historical data to project reimbursement and flag outliers. These insights help revenue cycle managers make data-driven improvements to processes.
Importantly, AI in medical coding is designed to augment human coders, not replace them. By handling the repetitive and straightforward tasks, AI allows coding staff to focus on complex cases that need clinical judgment. The result is a coder+AI team that is more productive and accurate than either alone, leading to significant gains in billing efficiency and reduced workload on staff.
Case Studies: AI Boosting Revenue Cycle Performance
Real-world deployments of AI in coding and billing have demonstrated clear benefits. Below are two examples highlighting improvements achieved with AI in medical coding and RCM:
Large Hospital Network: After implementing an AI-assisted coding system, a multi-hospital network reported a 30% reduction in coding time for its billing staff. Coding accuracy improved by about 20%, as the AI caught errors and omissions that humans missed. By coding more comprehensively (capturing all applicable diagnoses/procedures), the hospital saw a 15% increase in appropriate reimbursements because fewer services were left unbilled (How AI is Improving Medical Coding Accuracy and Efficiency | Medwave). This network also noted that claims moved through the billing cycle faster, reducing days in A/R and improving cash flow.
Outpatient Clinic Group: A group of specialty clinics deployed an AI coding and billing solution and saw claim performance metrics rise sharply. Claim denials dropped by 40% due to more accurate coding and proactive correction of errors before submission. Billing turnaround time sped up by about 25% (How AI is Improving Medical Coding Accuracy and Efficiency | Medwave) – what used to take days now took hours, on average. Coders and billers in the clinics reported higher job satisfaction, as the AI handled tedious tasks (like pulling data from EHR notes), allowing staff to concentrate on exception cases and patient-focused tasks (How AI is Improving Medical Coding Accuracy and Efficiency | Medwave). These improvements led to higher net collections for the clinics without adding staff, essentially boosting productivity and revenue simultaneously.
Other case studies echo similar results – from community hospitals using NLP in healthcare coding to capture complications and comorbidities (thereby earning appropriate DRG payments), to large health systems that integrated AI for prior-auth checks and saw faster approvals. Across the board, AI in revenue cycle management has shown it can reduce claim denials, speed up reimbursements, and increase the yield of each claim.
Challenges in Adopting AI for Medical Coding and Billing
While the advantages of AI are compelling, healthcare organizations face several challenges when implementing AI-driven coding solutions. Key hurdles include:
Integration with EHR and workflows: Incorporating AI into existing electronic health record (EHR) systems and billing workflows can be complex. Hospitals often use legacy EHRs or multiple software systems. The AI tool must integrate seamlessly – pulling clinical notes, inserting codes or flags, and interfacing with billing modules. Ensuring smooth system integration and minimal disruption to current processes is a significant technical and organizational challenge (How AI is Improving Medical Coding Accuracy and Efficiency | Medwave). Many providers start with pilot programs to fine-tune integration before full rollout.
Data privacy and security: Healthcare data privacy is paramount, and AI systems need vast amounts of patient information to train and operate. Organizations must ensure any AI-driven coding platform is fully HIPAA-compliant and secure. This means implementing encryption, strict user access controls, and regular security audits for the AI system (Technological Advances in Medical Coding). A breach or misuse of sensitive patient data not only undermines trust but could lead to legal penalties. Healthcare providers also need clarity on data ownership – if using a vendor’s AI, patient data must be handled in accordance with privacy regulations and contracts.
Regulatory and coding compliance: AI recommendations must adhere to all coding guidelines, payer rules, and regulations. Compliance is a major concern – for example, if an AI upcodes (assigns a higher-paying code improperly) or violates Medicare coding rules, the provider is still liable. Thus, AI outputs require oversight, and systems need thorough validation. Regulatory bodies like CMS have not issued specific guidelines on AI in coding yet, but standard requirements (accurate, audit-able coding) apply. Ensuring the AI’s decisions can be explained and justified with documentation is critical. In short, AI must support coding compliance rather than inadvertently create compliance risks (How AI is Improving Medical Coding Accuracy and Efficiency | Medwave).
Workforce training and acceptance: Adopting AI tools means staff must be trained to use new software and adapt to modified workflows. There can be resistance to change among coders and billers used to manual processes (Technological Advances in Medical Coding). It’s important to communicate that AI will assist, not replace, human expertise. Comprehensive training and change management are needed so staff trust the AI recommendations and know how to handle exceptions. Over time, as they see reduced workload and better results, acceptance grows – but the transition can be a challenge initially.
Upfront costs and ROI concerns: Implementing AI for RCM often requires a sizable investment in software licenses, IT integration, and training. Smaller practices may find the initial cost prohibitive (Technological Advances in Medical Coding). Decision-makers must weigh these costs against the expected ROI. Fortunately, many early adopters report that the long-term gains (higher collections, lower labor costs) offset the upfront expense (Technological Advances in Medical Coding). Still, securing budget and demonstrating ROI to leadership remains a hurdle in adopting new AI technologies.
Despite these challenges, the trend is toward overcoming them as AI matures. Vendors are improving the interoperability of AI solutions, and success stories of safe, compliant AI deployments are easing fears. With proper planning around integration, privacy, and compliance, healthcare organizations can reap the benefits of AI in coding while managing the risks.
Market Outlook and ROI for AI-Driven Revenue Cycle Management
The market for AI-driven revenue cycle management in healthcare is growing rapidly as providers seek to automate and optimize billing. AI in medical coding and billing is no longer experimental – it’s becoming a mainstream strategy for healthcare financial improvement.
(AI In Revenue Cycle Management Market Size, Report by 2034) Market projections for AI in revenue cycle management show robust growth.
Strong market growth: Recent industry analyses project the AI in medical coding market to expand at roughly 13% CAGR through the next decade. It was valued around $2.6 billion in 2024 and is forecasted to reach about $9 billion by 2034 (AI In Medical Coding Market Size To Worth USD 9.16 Bn By 2034) (AI In Medical Coding Market Size To Worth USD 9.16 Bn By 2034). This growth is fueled by hospitals and physician groups investing in AI solutions to curb rising administrative costs and claim denials. In fact, nearly all healthcare leaders anticipate adopting AI in RCM – one survey found 98% of health system leaders expected to use AI in revenue cycle management by 2023 (AI In Revenue Cycle Management Market Size, Report by 2034). The RCM software market as a whole is also growing (over $80+ billion by late 2020s), with AI-driven tools accounting for an increasing share of that spending.
ROI and performance improvements: Early adopters of AI in RCM are reporting tangible returns on investment. In a 2025 industry-wide survey by Black Book Research, 83% of healthcare organizations said AI-driven automation reduced claim denials by at least 10% within 6 months ( Black Book Research Releases First Industry-Wide Evaluation of AI-Driven Revenue Cycle Management Solutions | Newswire ). Moreover, 68% of RCM executives saw improved net collections after implementing AI, and 39% reported a cash flow increase of over 10% within six months ( Black Book Research Releases First Industry-Wide Evaluation of AI-Driven Revenue Cycle Management Solutions | Newswire ). Such quick wins suggest a fast payback period for AI investments. By improving collection rates and cutting denial rework, AI tools directly contribute to revenue uplift. They also reduce manual labor – increasing productivity per full-time employee – which lowers operational costs. All these factors drive a compelling ROI. It’s not uncommon for a hospital to recoup its AI implementation costs in under a year thanks to the boost in recovered revenue and efficiency.
Future outlook: The coming years will likely see broader AI adoption in coding and billing, including more advanced AI (e.g. generative AI) to assist with clinical documentation improvement, automated patient eligibility checks, and predictive analytics for financial risk. Market growth is also spurred by improvements in AI technology itself, which is becoming more accurate and easier to integrate. As vendors and health systems navigate data privacy and compliance successfully, confidence in AI-driven RCM will continue to rise. The expected result is a virtuous cycle: greater adoption leads to better AI models and lower costs, which in turn leads to even more adoption.
Healthcare organizations that embrace AI in medical coding and billing stand to gain significant competitive advantages – from higher billing efficiency and lower denial rates to more robust financial performance. With the healthcare industry under pressure to do more with less, AI-driven revenue cycle management offers a timely solution to capture revenue that might otherwise be lost to inefficiency.
Sources:
Poland, L., & Harihara, S. Claims Denials: A Step-by-Step Approach to Resolution. Journal of AHIMA (2022) – Denial rates ~10%+, 20% of claims denied industry-wide, 60% not resubmitted ( Claims Denials: A Step-by-Step Approach to Resolution ); cost to rework denials ( Claims Denials: A Step-by-Step Approach to Resolution ); avg. $5M annual loss from denials ( Claims Denials: A Step-by-Step Approach to Resolution ).
Lagasse, J. Commercial payers delay paying out claims to providers. Healthcare Finance News (2023) – 31% of inpatient commercial claims >3 months to pay (vs 12% Medicare) (Commercial payers delay paying out claims to providers | Healthcare Finance News); similar outpatient delay stats (Commercial payers delay paying out claims to providers | Healthcare Finance News); certain denial types delay 45+ days (Commercial payers delay paying out claims to providers | Healthcare Finance News).
Taylor, L. Stop living in denials – The impact of inefficient billing processes. Physicians Practice (Nov 2023) – Insurers deny ~10–20% of claims (Stop living in denials – The impact of inefficient billing processes); 86% of denials avoidable, yet 65% of denied claims not resubmitted (Stop living in denials – The impact of inefficient billing processes); tech-enabled RCM benefits (shorter cycles, less A/R days) (Stop living in denials – The impact of inefficient billing processes).
The True Costs of Coding Errors: How AI Turns Challenges Into Opportunities. Billing-Coding.com (Sep 2024) – $82.7B spent on admin tasks (2022) ( The True Costs of Coding Errors: How AI Turns Challenges Into Opportunities ); $10.6B wasted annually on denial management ( The True Costs of Coding Errors: How AI Turns Challenges Into Opportunities ); AMA: 11% of claims denied in 2022 (~110k claims for avg. system) ( The True Costs of Coding Errors: How AI Turns Challenges Into Opportunities ).
How AI is Improving Medical Coding Accuracy and Efficiency. Medwave (Sept 2024) – Case studies: 30% faster coding, 20% higher accuracy, +15% reimbursements (hospital) (How AI is Improving Medical Coding Accuracy and Efficiency | Medwave); 40% fewer denials, 25% faster billing (clinic group) (How AI is Improving Medical Coding Accuracy and Efficiency | Medwave); notes challenges of data quality, integration, compliance (How AI is Improving Medical Coding Accuracy and Efficiency | Medwave).
Technological Advances in Medical Coding. Practolytics (2025) – AI in coding overview (Technological Advances in Medical Coding) (Technological Advances in Medical Coding); need for HIPAA compliance and data security (Technological Advances in Medical Coding); initial cost vs. long-term benefit (Technological Advances in Medical Coding); importance of training and change management (Technological Advances in Medical Coding).
Black Book 2025 AI RCM Survey Press Release. Newswire (Feb 2025) – 83% saw >10% denial reduction with AI in 6 months ( Black Book Research Releases First Industry-Wide Evaluation of AI-Driven Revenue Cycle Management Solutions | Newswire ); 68% improved collections, 39% saw >10% cash flow gain ( Black Book Research Releases First Industry-Wide Evaluation of AI-Driven Revenue Cycle Management Solutions | Newswire ); 96% say AI analytics improve long-term RCM strategy ( Black Book Research Releases First Industry-Wide Evaluation of AI-Driven Revenue Cycle Management Solutions | Newswire ).
AI in Medical Coding Market Size, 2024–2034. Precedence Research (Oct 2024) – Market size $2.63B in 2024 to $9.16B by 2034 (CAGR ~13.3%) (AI In Medical Coding Market Size To Worth USD 9.16 Bn By 2034) (AI In Medical Coding Market Size To Worth USD 9.16 Bn By 2034); high adoption expected with nearly all leaders planning AI in RCM (AI In Revenue Cycle Management Market Size, Report by 2034).